The need for regularly updated and accurate geospatial data has grown exponentially in the last decades. Mobile mapping LiDAR data serve as an important source for various applications, including outdoor 3D modelling, generation of geographic information system (GIS) data, infrastructure mapping and autonomous driving.
At Flai, innovation is at the heart of everything we do, and today, we are thrilled to introduce our latest classifier: the FlaiNet mobile mapping classifier.
This deep learning algorithm automates the classification of LiDAR point clouds in mobile mapping into 19 distinct categories, offering new possibilities for industries dependent on precise spatial data.
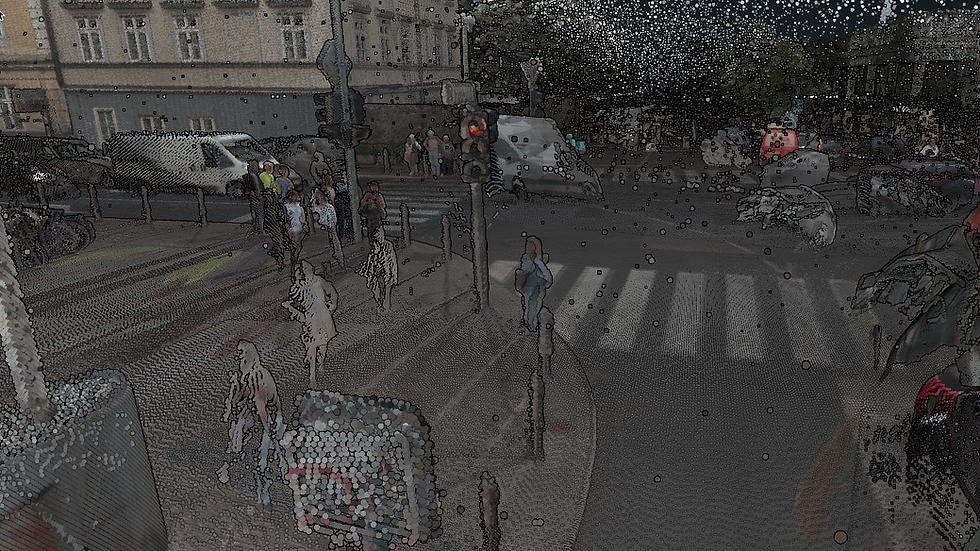
Our mobile mapping classifier takes a raw LiDAR point cloud as input and automatically assigns each point to one of 19 semantic categories:
Roads
Sidewalks
Traffic Islands
Pedestrian Crossings
Buildings
Trees
Other Vegetation
Traffic Lights
Traffic Signs
Wires
Pedestrians
Vehicles
And more...
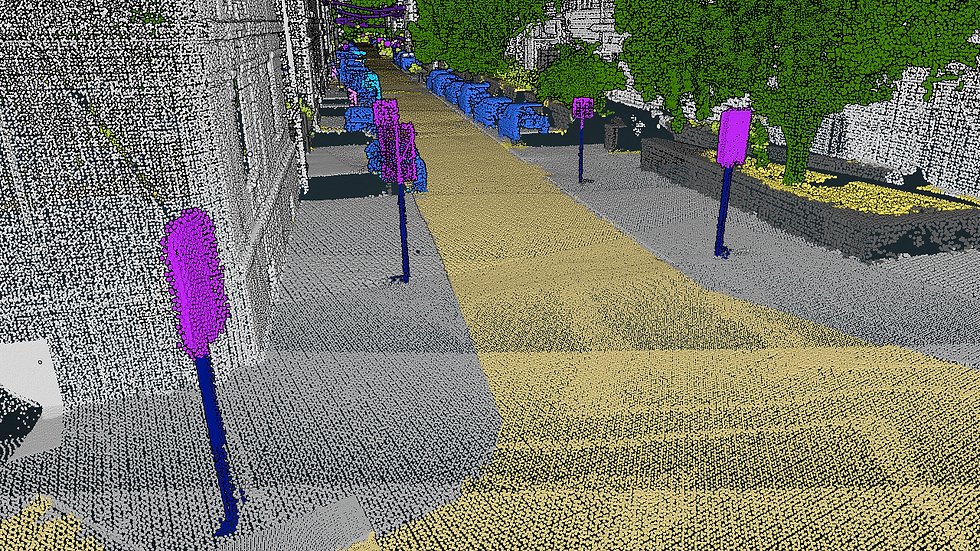
You can classify point clouds directly on the Flai web application. Simply create a classification flow consisting of a point cloud reader, a FlaiNet mobile mapping classifier and a point cloud writer. Run the flow and wait until you get an email notification that the dataset is ready for viewing. For additional information make sure to read our blog post on how to create and run a processing flow.

To validate our newest FlaiNet mobile mapping cassifier we processed 1 square kilometre of mobile mapping data of the Slovenian capital Ljubljana. The dataset consisted of 5 billion points, with an average of 5500 points per square meter.
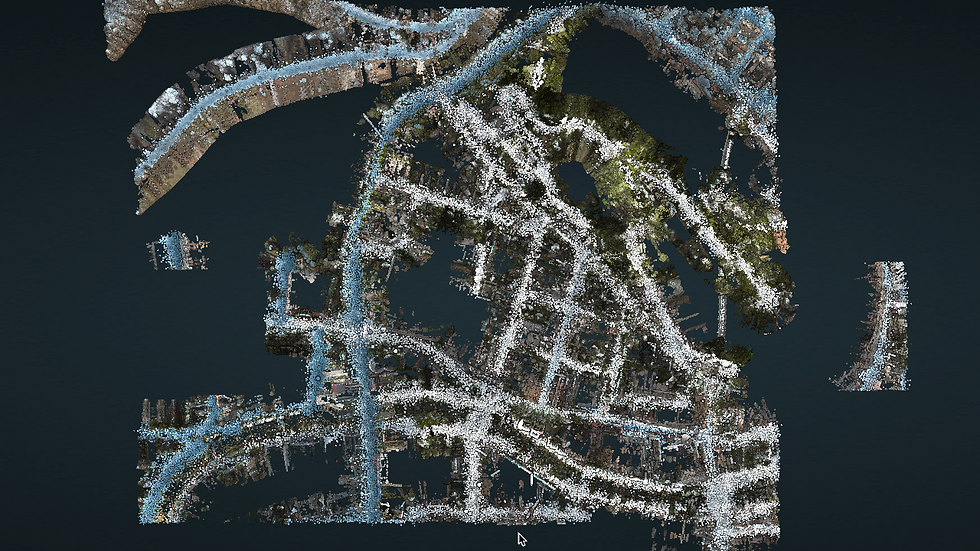
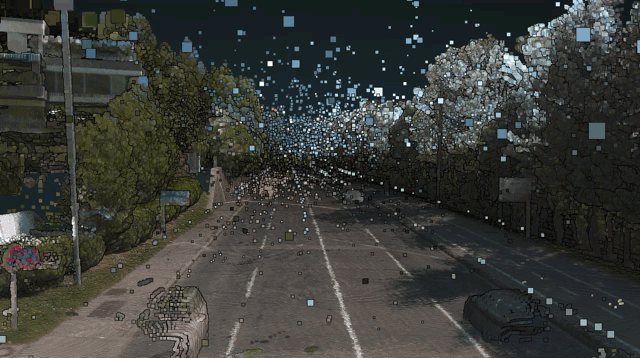
Flai's mobile mapping classifier marks a significant leap in automating the classification of LiDAR point clouds in mobile mapping, categorizing them into 19 crucial semantic groups such as roads, buildings, trees, and traffic signs. Overcoming challenges posed by mobile mapping datasets, including moving vehicles and noise, this classifier provides a streamlined solution.
The FlaiNet mobile mapping classifier unlocks a realm of possibilities, enabling industries to make informed decisions, derive valuable insights, and enhance operational efficiency based on the classified point clouds.
Comments